Last month we released v1.0.0 of the Overture schema and “general availability” versions of four themes:
base, buildings, divisions, and places. Our addresses theme launched in alpha with 200 million addresses
for 14 countries; we anticipate adding more address data each month, country by country, starting with
Switzerland in the August release.
The transportation theme remains in beta and we expect to make significant changes over the next couple
months. In this post, we'll give you a preview of three key improvements to the transportation schema in the August
release, which will be available next week.
Transportation schema changes
The schema changes we plan to implement over the next few releases will better support routing and map
display use cases, and make it easier for our users to integrate Overture transportation data into their
pipelines and products. These improvements are necessary if we are to achieve our goal of a GA release in October.
Here are the steps we're taking in the August release to move us toward that goal.
Subclasses
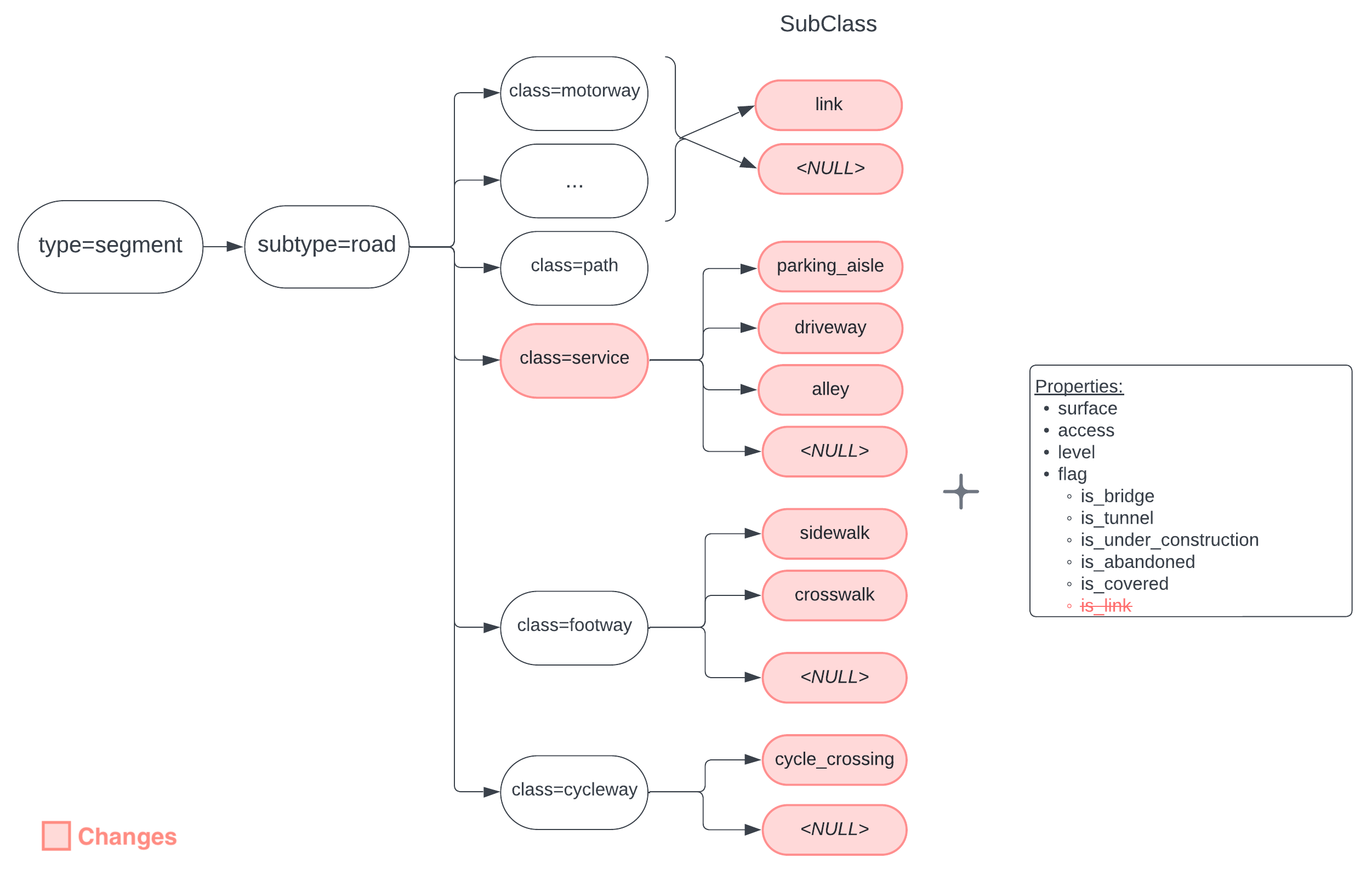 |
---|
A diagram of the new class and subclass structures |
We added a new subclass
property that refines the descriptions of segments by:
- clearly specifying the usage of a length of road, and using linear referencing instead of further segmentation to pinpoint a change in how the road is used
- properly classifying detailed information from OpenStreetMap
Subclasses require non-overlapping geometries, which will make it easier for routing engines to consume our
road data and generate turn-by-turn directions. The new class and subclass structures allow us to capture more
information about roads from our data sources. For example, we’ve been able
to move more than 30 million “unknown” road segments from OSM into the new subclasses, laying the groundwork
for richer and more detailed map displays with Overture data.
Here's how class, subclasses, and flags are modeled in the Overture schema:
class: footway
subclass_rules:
- value: sidewalk
between: [0, 0.6]
- value: crosswalk
between: [0.6, 1.0]
road_flags:
- values: [is_bridge]
between: [0.2, 0.3]
- values: [is_under_construction]
between: [0.3, 0.5]
class: service
subclass_rules:
- value: driveway
between: [0, 0.5]
class: primary
subclass: link
subclass_rules:
- value: link
Connectors
To better support routing use cases, we added to the segment feature type a new property called
connectors
: an array of IDs with pre-computed linear reference values that explicitly link segments and
connector features via coordinates. The connectors
property contains a list of connector features to which a segment feature is physically connected and the relative location of those connector features along the segment. Each connector is a possible routing decision point, meaning it defines a place along the segment in which there is possibility to transition to other segments that share the same connector.
This new property — a replacement for the existing connector_ids
property — will reduce complexity and improve accuracy as we calculate the intersections between segment and connector features. We plan to deprecate the connector_ids
property in the August release and remove it from the schema in the October release.
Here are examples from the Overture schema comparing the new and deprecated features:
- new connectors property
- deprecated connector_ids property
id: overture:transportation:segment:123
type: Feature
geometry:
type: LineString
coordinates: [[0, 0], [0.03, 0], [0.10, 0]]
properties:
theme: transportation
type: segment
version: 1
subtype: road
class: secondary
connectors:
- connector_id: fooConnector
at: 0
- connector_id: barConnector
at: 0.3
- connector_id: bazConnector
at: 1
road_surface:
- value: paved
id: overture:transportation:segment:123
type: Feature
geometry:
type: LineString
coordinates: [[0, 0], [0.03, 0], [0.10, 0]]
properties:
theme: transportation
type: segment
version: 1
subtype: road
class: secondary
connector_ids: [fooConnector, barConnector, bazConnector]
road_surface:
- value: paved
Destinations
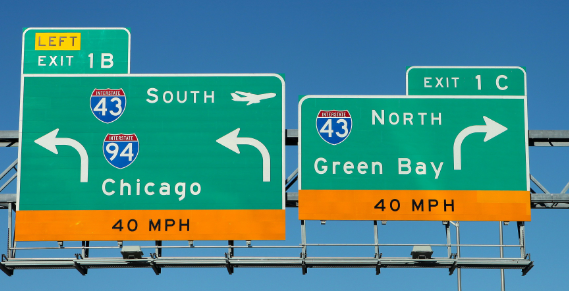 |
---|
Highway signs help us get from point A to Green Bay |
Also in support of routing use cases, we added a destinations
property to the segment feature type.
This property describes the transitions from one segment to another on the way to a specified location.
In turn-by-turn routing applications, this is what is known as “follow signs for” — the human-readable
directions and signposts along a road, highway, or interstate that get us from point A to point Z, by way
of any number of paths in between. We designed destinations
with a flexible schema that will allow us to
capture and model navigation data from many different sources.
Here's an example of how highway sign information in the picture above is modeled in the destinations
schema:
destinations:
- when:
heading: forward
from_connector_id: 08f274370d59a8840436ff3bad31c60e
to_connector_id: 08f274370d59a8840436ff3bad31c60e
to_segment_id: 08a274370d59ffff043fff3b802d7aa5
final_heading: forward
labels:
- value: Green Bay
type: unknown
- value: I 43 North
type: route_ref
symbols:
- NULL
Recently we made our transportation splitter tool
available in a public GitHub repository. The tool is an experimental PySpark script and Jupyter notebook that offers
multiple ways to split Overture’s transportation features into smaller and simpler segments. Our new transportation guide includes several examples of how to use it. Please
take it for a test drive and let us know what you think!